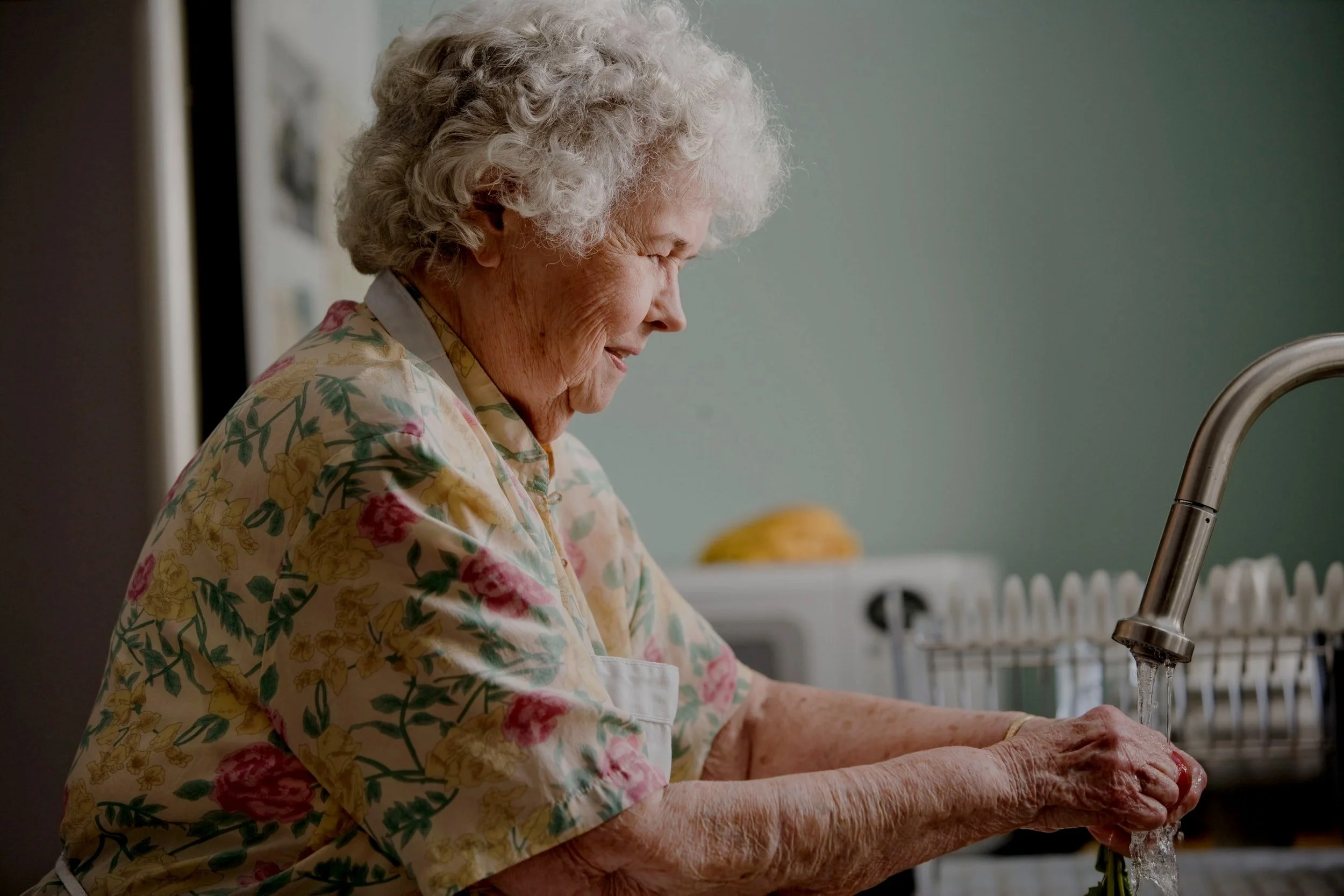
Facilitating Aging-In-Place with Technology
A remote, unmoderated feasibility study using biomedical sensors to try to passively detect cognitive impairment.
-
Organization
Oregon Health & Science University
-
My Role
Research Associate
-
Tasks
Experimental Design, Data Collection and Management, Neuropsychological Testing and Scoring, Participant Recruitment and Coordination
Research Question:
Can we passively detect age-related cognitive change in a senior’s own home?
Result:
Yes.
Why does this matter?
Diagnosis of cognitive decline usually happens too late - after behavioral changes have become visible to family members or a doctor at an annual check-up.
Intervening at the beginning of decline offers a better chance of slowing of symptom progression and living independently for longer.
Stakeholders
To answer our research question, we needed to balance resources and needs from several different contributing groups:
The National Institute of Health and National Institute of Aging invested millions of dollars in this project and its ability to inform dementia treatment globally.
Expectations: Publications, rigorous primary research
Continuing care and eldercare organizations in Portland working to facilitate aging-in-place know that our research might reduce medical intervention for their community members, and have offered unprecedented access to their campuses and populations.
Expectations: Improved diagnostic accuracy, kind regard for their residents who are volunteering
Health-Tech companies (such as VTech) working to develop new technology that promotes independent functioning have partnered with us to test new products in our community lab.
Expectations: Development and testing of health-tech prototypes that can be patented and distributed widely
Assumptions
We started with several baseline assumptions mined from previously published research:
Age-related cognitive change currently affects at least 1 in 10 seniors.
The senior population (age 65+) is rapidly rising in percentage of American population, which will require innovation in resources to accommodate geriatric medical issues.
Age-related cognitive changes are often diagnosed only when functional impairment occurs due to stigma, lack of self-awareness, limited contact with medical providers, and other factors.
Early identification and intervention is key - dementia is notoriously difficult to reverse - most treatment aims only to slow the progression.
Hypothesis
We can identify age-related cognitive changes earlier by creating an unobtrusive, remote system that collects data continuously as a senior moves through their day.
We developed this hypothesis based on literature reviews and competitive analysis of existing products, working to push the boundaries on what might be possible to achieve via remote, automated data collection in the field.
Methodology
We designed a system of in-home sensors to collect multiple ongoing data streams via various remote, automated tools.
It was important that these tools were accurate, easy to use and fix, and not disruptive to the homeowner.
-
Infrared movement sensors installed around the home collect room-to-room transitions, walking speed, and front door entry/exits.
Changes in movement patterns have been shown to be correlated with cognitive decline.Time out of house has been shown to be a measure of social engagement and is often correlated with loneliness.
-
A smart scale and digital pill box allow for additional passive data collection.
Self-report of medication adherence is notoriously spotty in those with cognitive decline, so our pill box sends continuous data about time and frequency of adherence.
The smart scale measures changes in pulse, body composition metrics, pulse wave velocity, environmental temperature, and ambient carbon dioxide level can be indicators of changes in health, which can also be related to cognitive decline.
-
A personal computer installed in the home allows us to collect both attitudinal and quantitative behavioral data.
Using the computer to launch frequent, short questionnaires about mood, health and life events can offer context for changes in the continuous data, and mitigate self-report issues that may come up at less frequent medical visits.
Passive quantitative behavioral data such as mouse clicks and response times can be collected as well.
-
The gold-standard for assessing cognitive decline is annual, in-person neuropsychological testing. We collected this data manually to compare with the continuous remote sensor data and to provide a baseline for validation.
Usability Testing
We tested usability in several different ways, conducting both generative and evaluative testing to determine which components had the right balance of importance and feasibility to continue investing in.
Since its genesis, the sensor platform has evolved considerably as we gathered data from users and pushed the limits of available technology.
-
Surveys
A range of standardized and specific-to-this-project periodic questionnaires evaluating usability and pain-points.
-
Focus Groups
Working with prospective and active users to hear their experience with the platform has been invaluable.
-
Prototype Testing
We built out a prototype platform in a lab that mimics a studio apartment, allowing us to test and validate each tech before launch.
-
Launch and Test
Beginning over a decade ago, we began launching platforms in volunteers’ homes. As technology has improved, research questions evolved, and we received feedback from our users, we’ve iterated our design.
Results
Platform Launch Success!
Beginning with only a handful of volunteers who offered their homes to us in the early years of prototyping, we’ve now installed this vetted platform in hundreds of homes in the Portland metro area, and have been collecting data continuously and analyzing findings for over a decade.
Individual users of this platform have been able to receive early diagnosis and intervention when appropriate.
This longitudinal data set is now a deeply valuable resource available to other researchers for analysis or collaboration here.
Analysis Examples
There are now many published papers using data from this platform.
Here are some examples of how data collected in this way can illustrate subtle behavioral changes that may otherwise be missed:
Walking Speed Variability
We know that walking speed becomes more variable with onset of cognitive impairment. We were successfully able to show subtle changes in gait over time using our “walking lines”, or a set of infrared sensors that measures walking speed many times a day for each participant.
Groups with different walking speed patterns (and therefore different cognitive functioning) were distinguished using latent trajectory models.
In this figure, Group 1 corresponds to early-stage mild cognitive impairment, validated by neuropsychological testing. We can see that overtime, walking speed becomes more variable as cognitive decline deepens.
Life Event Visualization
This spiral plot shows data from sensors measuring room-to-room transitions. The figure allows you to see patterns of movement throughout the day and over the course of 6 months: Each 24-hour day is a ring, and each color represents a room.
For instance, at about days 75-85 on this plot, you can see a band of pink dots, indicating increased kitchen usage at mealtimes. This corresponds with a Norovirus outbreak at the continuing care community where this data was collected, showing that changes in expected movement patterns are visually identifiable.
Other uses for this type of data might be to see if night time waking is increasing over time, or routinized tasks are becoming more variable - both potential indicators of cognitive decline.
Benefits of the Platform
Years of data collection and analysis have allowed us to validate the platform’s strengths:
It allows researchers to improve study outcomes' objectivity and reliability via passive validation of self-report data.
We can reduce sample sizes and decrease time to answer due to the high granularity of data.
We’ve uncovered hidden adverse events that can be subtly detected, but might have gone unreported by the participant.
The platform facilitates continuous post-launch assessment of a target intervention or tool, allowing us to contract with health-tech organizations to test their new products.
Ongoing Considerations
We’ve learned a lot in the years of implementing this platform:
We need to ensure that our volunteers understand the protocol and are comfortable with the nature of this research. Generating participant buy-in promotes adherence and ongoing communication throughout the project.
The platform was designed with open-source software or accessible APIs to allow for replication. It is created to be technology “agnostic” to allow for a wide variety of technology to be “plugged in” as needed, which has proven necessary as we expand the suite of products tested.
We prioritized a “low-touch” design that would need minimal interference to continue collecting data. Remote, daily, digital “check-ins” on the health of the system in each home are run, and technologists only occasionally need to visit the home to repair failing components.
My Role
As a Research Associate, my role in managing this platform consisted of:
Recruiting participants via presentations and focus groups
Conducting neuropsychological batteries with participants annually to validate data collection
Transitioning the study to digital data collection forms (the study had been started prior to this being an easy task)
Writing an extensive paper detailing the methodology of the lab to facilitate replication elsewhere
Providing internet and technical support both remotely and in-home for participants as components of the platform needed repair or updating
Next Steps
The strength in this platform lies in its flexibility now that it has been established widely. It functions both to evaluate changes in movement or behavior patterns in a single person over time, or as a broad testing ground for evaluating new health technology.
Establishing more communities of “Living Labs” in this way could diminish the existing chasm between technology design intention in the lab and functionality in the field, creating more opportunities to understand the needs of an aging population, and reducing iteration and time to market for tools that can facilitate aging in place.